RESEARCH
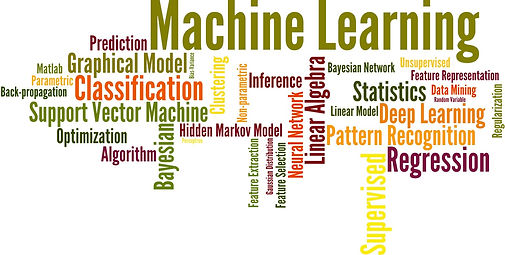
MACHINE LEARNING and DEEP LEARNING
-
Our major interests are to develop biologically plausible machine learning algorithms for various applications to brain and cognitive engineering including braing-computer interfaces and brain disease diagnosis.
-
We design deep architectures for heterogeneous modality fusion and developing learning algorithms, possibly even with a small number of samples as a human brain does.
-
We develop deep probabilistic state-space models that can discover the dynamic patterns in observations and represent knowledge in a hierarchical and interpretable manner.
-
We are also interested in devising computational models, e.g., deep learning, dynamic state-space model, sparse regression model, by which target-related features can be selected in a self-taught manner, and thus we can enhance task performances.
-
Designing deep architecture and developing learning algorithms are important issues to discover latent or abstract feature representations for better understanding and investiation of the underpinning phenomena in observations.
BRAIN DISORDER DIAGNOSIS or PROGNOSIS
-
For an expert system of brain disorder diagnosis or prognosis, we aim to develop novel methods that can integrate multiple neuroimaging modalities, e.g., MRI, PET, fMRI, and genetic data, so that we maximally utilize the complimentary information inherent in different modalities.
-
We develop algorithms that systematically fuse heterogeneous modalities of sMRI, PET, fMRI, etc. for better use of complementary information, and thus to identify neuroimaging biomarkers.
-
We develop machine-learning algorithms that can estimate the underlying functional patterns from fMRI images and utilize the estimated information for disease diagnosis/prognosis.
-
The brain disorders of our interests include Alzheimer's Disease (AD) and its prodromal stage Mild Cognitive Impairment (MCI), Autism Spectral Disorder (ASD), and schizophrenia.
-
The functional connectivity estimation based on resting-state fMRI is also one of our interests for better understanding of brain mechanisms in information processing, and its application to brain disorder diagnosis or prognosis.
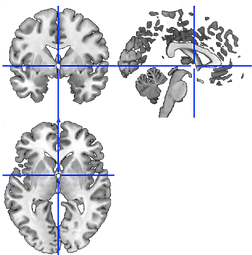
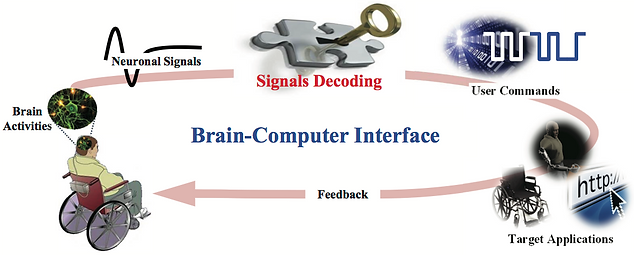
NON-INVASIVE BRAIN-COMPUTER INTERFACES
-
For practical applications of Brain-Computer Interfaces (BCIs), we focus on developing advanced signal prosessing and machine learning algorithims; spatio-spectral filter optimization and transfer learning, to name a few.
-
We developing a fully automated learning system by optimizing spatial-spectral filters, by which we can discover intention-related physiological patterns.
-
We develop BCI-oriented but paradigm-independent algorithms that intelligently remove artifacts/noise and learn a user-specific brain signal patterns by utilizing their resting-state signals.
-
We are interested in designing various experimental paradigms to understand the physiological patterns and developing user-friedly interfaces by efficiently utilizing such patterns.
-
In combination with other neuroimaging modality such as Near Infra-Red Spectrography (NIRS), we investigate functional connectivies and their characteristics in a human brain.